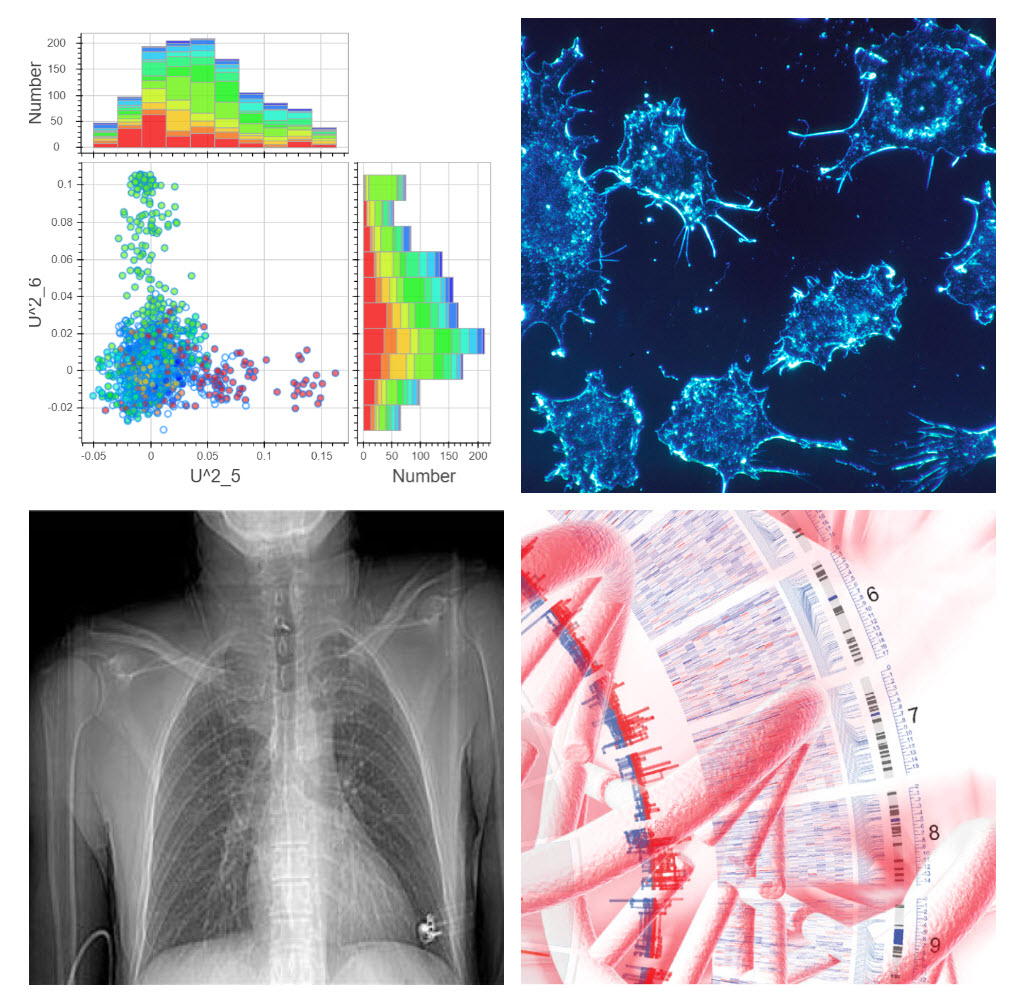
2019 TEAMS
TEAM 1: Hippocampal oscillatory patterns during associate recognition memory
Investigate hippocampal oscillatory patterns to test predictions of dual process theory to classify recognition versus recollection using memory retrieval data.
The goal of investigating hippocampal oscillatory patterns to test predictions of dual process theory motivated us to apply the Associate Recognition (AR) paradigm to intracranial EEG patients with depth electrodes inserted into both cerebral hemispheres, including both the anterior and posterior hippocampus simultaneously. Twenty subjects with medication-resistant epilepsy who underwent stereo-electroencephalography (sEEG) surgery with the goal of identifying their ictal onset region(s) performed AR paradigm during their monitoring period at UT Southwestern. Overall, there were 69 anterior and 41 posterior hippocampal electrodes included in the dataset. The subjects view series of word pairs during the encoding (study) phase. During the retrieval (test) phase, the word pairs were shown as intact (studied together), rearranged (both words were studies but on different trials), and new (neither word was studied) for two seconds, then received a cue to respond their decision whether the word pair was intact or rearranged or new. The sEEG signal was sampled at 1 kHz during acquisition and down sampled at 500 Hz offline for processing. Line noise was notch filtered and a kurtosis algorithm (with threshold of 4) was used to exclude abnormal events and interictal activity. The power and phase values (58 frequencies and 900 time steps) were extracted from 1800 ms time windows following the appearance of the study item using Morlet wavelets. We aim to:
1) Classify word pairs as either recollected or recognized using encoding signal and compare performance to the classification using retrieval signal.
2) Determine which features are most critical for classification.
3) Use classifier trained on encoding data to classify recognition versus recollection using retrieval data (measure of reinstatement of information).
Classifier can be trained across subjects/electrodes (including anterior and posterior or hemisphere) to increase the number of trials available for classification.
Team Leads: Bradley Lega, MD and Srinivas Kota, PhD, Neurosurgery, https://www.utsouthwestern.edu/labs/tcm/
team 2: A Deep convolutional neural network for identifying thyroid cancer
Develop a more accurate system for identifying thyroid cancer by applying a deep convolutional neural network algorithm to data from a UTSW thyroid cancer database.
Differentiating benign thyroid nodules from cancer is a significant health challenge, as thyroid nodules are present by ultrasound (US) in a staggering 19 – 35% of the general adult population (approximately 130 million people). Most of these nodules are benign, however the diagnostic process is costly, inefficient, and leads to unnecessary surgery and morbidity. We propose to develop a more accurate system for identifying thyroid cancer by applying a deep convolutional neural network (DCNN) algorithm to data from our thyroid cancer database, which includes a large number patients who have been followed clinically for several years. We propose to develop a new risk prediction and prognosis tool to predict the individualized risk of death and recurrence from thyroid cancer. We envision two modes of analysis; 1) exploratory analysis, and 2) clinical performance optimization. In the exploratory mode we will leverage advanced unsupervised machine learning techniques such as manifold learning and dimension reduction (DR) to visualize multi-modal, integrated, high-dimensional data (as a point cloud). We hope to build an interactive, GUI-based data visualization dashboard to allow rapid traversal of this complex data by scientists and clinicians alike. Additionally, we will incorporate and overlay the proposed predictive models as they are developed and refined, and provide the ability to annotate data/models on the fly for subsequent use with other models. In the second mode, performance optimization, we will build on the recognition patterns discovered in the augmented data exploration, and then refine and optimize specific candidate predictive models emphasizing those with high potential for clinical impact/utility. The most high-performing predictive models will then be validated retrospectively and prospectively. At the conclusion of this project, we will have a deep learning tool that has improved ability to detect thyroid cancer in patients with thyroid nodules. We also anticipate that we will have a risk prediction tool that accurately identifies the risk of recurrence and death after initial treatment of thyroid cancer. Through this process, we will have established one of the first comprehensive annotated databases of thyroid ultrasound images that will allow development and validation of a deep learning algorithm to accurately differentiate benign from malignant thyroid nodules.
Team Lead: Fiemu Nwariaku, MD, Surgery, https://www.utsouthwestern.edu/labs/nwariaku/
Team 9: Real-Time Cardiac Assessment of Catheterization-Derived Fick and CMR-Derived Flow
Improve workflow, reliability and reproduciblity of interventional cardiac MRI through a web/app-based user-friendly display for real-time assessment.
Cardiologists are tasked with the role of determining complex hemodynamic information that is important to determine the need for catheter-based and/or surgical intervention. Today’s standard of care practice involves performing cardiac catheterization and cardiac magnetic resonance (CMR) separately. Catheterization by x-ray is used primarily to collect pressure and saturation data and to intervene on hemodynamically significant holes in the heart. In addition, interventionalists are able to place stents, coils, and percutaneous valves. Meanwhile, CMR is a powerful emerging tool to help cardiologists and CT surgeons answer complex physiology questions by showing very accurate function and flow data.
Interventional cardiac magnetic resonance (iCMR) is a new approach in congenital cardiology gaining traction around the world. In the US, the National Institutes of Health (NIH) and Children’s Health Dallas are the only two centers currently actively pursuing this research in the congenital heart population. iCMR is like a normal heart catheterization, but the procedure occurs in the MRI magnet instead of the catheterization X-ray lab. It is our hope that a radiation-free heart catheterization will become standard of care for patients in the future.
We would like to improve the workflow and ease of calculating important hemodynamic information derived by cardiac catheterization, CMR, and/or iCMR. These numbers are important because they will help guide therapy and are often what surgeons and cardiologists analyze to determine if intervention is necessary. Cardiac catheterization and CMR use different techniques to ultimately produce the same hemodynamic information. Both techniques use simple but unrelated equations to determine the patient’s systemic and pulmonary cardiac flows. The current workflow of using these equations is completely manual and subject to human error.
Our goal is to improve workflow, reliability, and reproducibility by improving the accessibility of these hemodynamic equations through a web/app-based user-friendly display that will allow the operator to obtain information in real time.
Team Lead: Yousef Arar, MD, MPH, Pediatric Cardiology, www.utsouthwestern.edu/education/medical-school/departments/pediatrics/divisions/cardiology/
Team 12: Rapid evaluation of statistical strategies for predicting interactions and information transfer between proteins
Rapidly organize, statistically describe, and filter data and evaluate the performance of alternative models for phylogenetic correction on a gold-standard test set of protein interaction data.
Protein-protein binding interactions, inter-protein allosteric regulation, and protein complex formation provide a foundation for communication in the cell. An ability to predict and understanding protein interactions at residue-level resolution is necessary to design precise mutagenesis experiments, engineer protein complex specificity, and introduce new regulation. A substantial body of work now suggests that protein sequence co-evolution has major potential for predicting protein interactions.
We hypothesize the present approaches are limited by substantial levels of phylogenetic noise and an emphasis on local residue contacts rather than distributed residue networks. We seek to extend our analysis to a larger test set of complexes and more fully explore alternative models for phylogenetic correction. The hackathon would provide an excellent opportunity to rapidly organize, statistically describe, and filter our initial data set of protein interactions and to evaluate the performance of this method (as well as variations around it) on a gold-standard test set of protein interaction data. Following the hackathon, we plan to extend the most successful version of the analysis genome-wide (across E. coli) to globally infer the protein interactome.
Team Lead: Kimberly Reynolds, PhD, Green Center for Systems Biology, reynolds-lab.net/